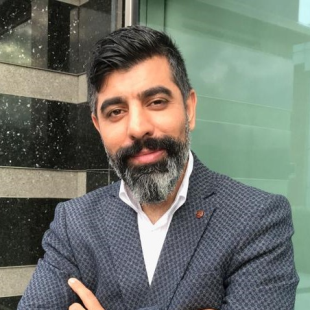
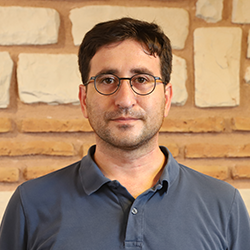
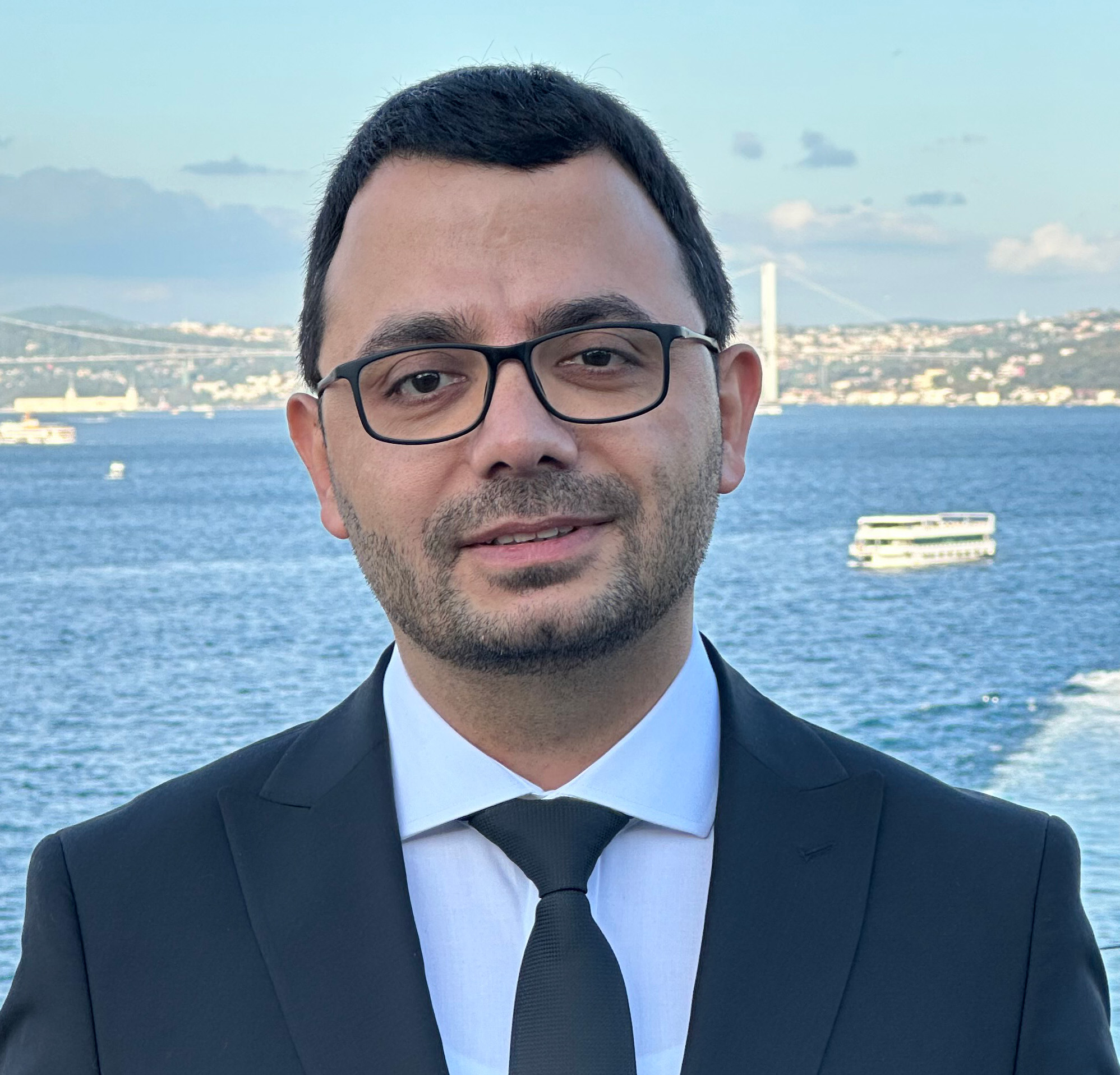
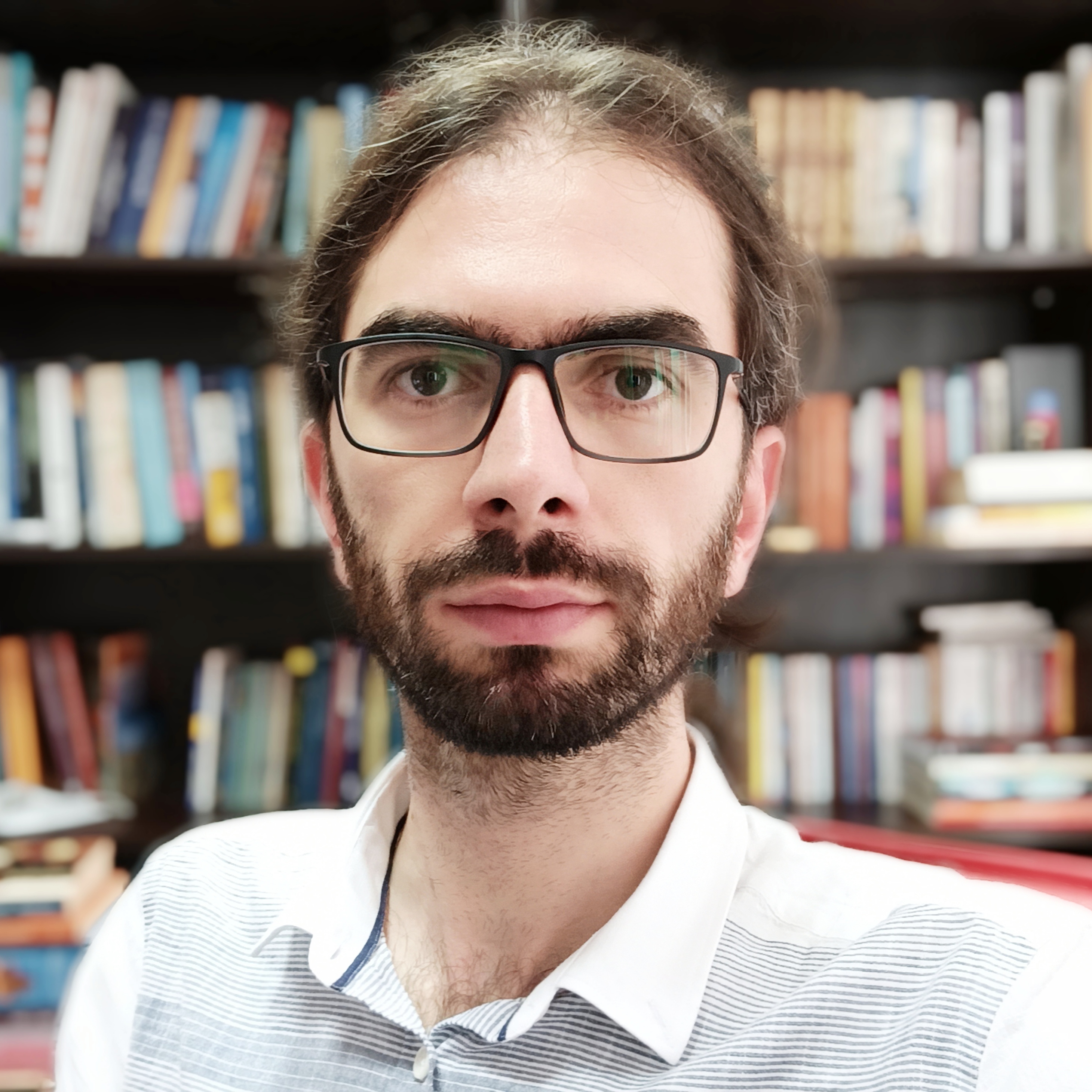
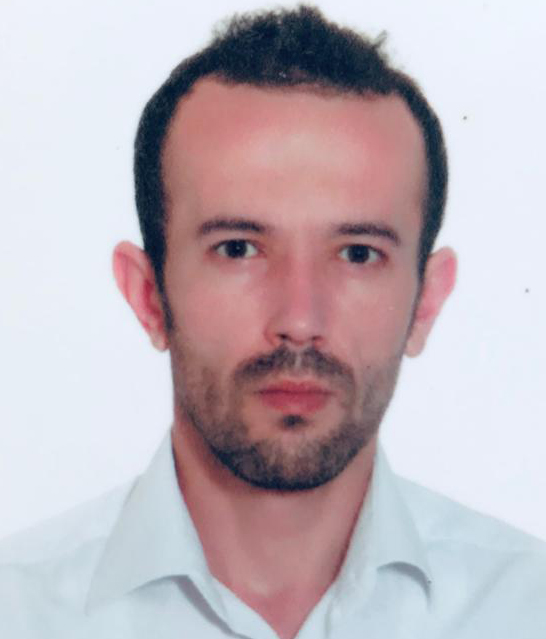
The City Development Index aims to compare cities objectively from a holistic perspective both at the international and national levels. In order for the City Development Index to have a strong methodology and healthy data infrastructure, a meticulous and intensive work process was carried out at every step in creating the index. The process of creating the City Development Index consists of the following steps: identifying the theoretical framework and key areas, data collection and indicator creation, determining the geographical scope, data processing, weighting and aggregation, classification and sequencing, and visualization and presentation.
When comparing the City Development Index internationally, a total of 48 indicators were constructed under 3 domains (social, economic, education/culture) and 12 dimensions. The dimensions of demographic dynamism, social welfare, health & safety, and environment have 18 indicators, while the dimensions of economic wealth, development, openness, and work life have 16 indicators. In the domain of education and culture, 14 indicators were produced under the dimensions of education, human capital, connectedness, and diversity and participation.
First, we identified 100 cities from each continent with populations of over 1 million and that had occurred the most in the indexes that had been examined within the scope of the study in order to compare the City Development Index internationally. Next, 50 cities were selected from each of these 100 cities by paying close attention to geographical inclusion and diversity. In particular, we selected cities that are prominent both in the selected sub-regions as well as in terms of country, paying attention to balancing the distribution of cities from among 5 continents (Africa, America, Asia, Europe, and Oceania) and 9 sub-regions (Africa, Western Europe, Eastern Europe, South America, North America, Middle East, South and Southeast Asia, Central and East Asia, and Oceania).
Macroform borders or the municipal borders of medium-sized cities/metropolitan areas or of the entire province of large cities have been taken as the basis while determining each city’s area for the international comparison. A total of 55 cities were compared by including 5 cities from Turkey with the 50 other cities being selected from around the world.
Cities Around the World Included in the City Development Index
The Indicator Structure of the City Development Index
DOMAIN |
DIMENSION |
INDICATOR |
INDICATOR CONSTRUCTION METHODS |
SOCIAL (18) |
Demographic |
Family Sustainability |
Ratio of crude marriage rate to crude divorce rate |
Population Reproduction |
Fertility rate per 1,000 women (aged 15-45) |
||
Life Expectancy |
Life expectancy at birth |
||
Infant Mortality |
Mortality rate per 1,000 live births |
||
Age Dependency |
Ratio of the population over the age of 65 to the active population |
||
Social Welfare |
Poverty |
Rate of population living under the national poverty line |
|
Automobile Ownership |
Number of automobiles per 1,000 people |
||
Cost of Living |
Average housing rental value (PPP) |
||
Comfortable Public Transportation |
Length of rail system(meters) per 1,000 people |
||
Health & Safety |
Access to Health |
Number of doctors and hospital beds per 1,000 people |
|
Traffic Accident Fatalities |
Number of deaths from traffic accidents per 100,000 people |
||
Work Safety |
Number of deaths from occupational accidents per 100,000 employees |
||
Suicide |
Number of suicides per 100,000 people |
||
Intentional homicides |
Number of homicides per 100,000 people |
||
Environment |
Recycling |
Recycling rate of municipal solid waste |
|
Air Quality |
PM2.5 ratio in the air |
||
Electricity Consumption |
Residential electricity consumption per capita (kWh) |
||
Water Consumption |
Residential water consumption per capita (m3) |
||
ECONOMIC (16) |
Economic |
GDP Per Capita |
Gross city product per capita (USA Dollars, PPP) |
Income Equality |
Gini coefficient |
||
Household Debt |
Ratio of household debt to GDP |
||
Inflation |
Average price increase (end of year) |
||
Development |
Investment |
Ratio of gross fixed capital formation to GDP |
|
Economic Transformation |
Share of tertiary sector in GDP |
||
Female Labor Force Participation |
Female labor force participation in the active population |
||
Taxation |
Ratio of collected taxes to GDP |
||
Openness |
Diversity in Work Life |
Percentage of foreigners in the labor force |
|
Foreign Direct Investment |
Ratio of foreign direct investment stock to GDP |
||
Economic Connection |
Ratio of total foreign trade to GDP |
||
Foreign Trade Balance |
Foreign trade balance |
||
Work Life |
Employment Structure |
Share of tertiary sector in employment |
|
Labor Force Participation |
Labor force participation rate in the population over 15 years of age |
||
Unemployment |
Unemployment rate |
||
Qualified Labor |
Percentage of the workforce with a university or higher-learning institution degree |
||
EDUCATION & CULTURE (14) |
Education |
Enrollment |
General enrollment rate |
Population in universities |
Percentage of higher education students in the population |
||
Quality of Education |
Number of students per teacher in primary and secondary schools |
||
Human Capital |
Population with Higher Education |
Educational attainment: Those aged 25+ with at least one post-secondary degree (%, cumulative) |
|
Number of Researchers |
Percentage of those working in R&D who are researchers |
||
R&D Expenditures |
R&D expenditures to GDP (%) |
||
Intellectual Property |
Number of intellectual property applications (patents, registered trademarks) per 100,000 people |
||
Connectedness |
Connectivity |
Ratio of the number of passengers within 80 km of the city airport(s) to the total population |
|
Attractivity |
Number of overnight tourists |
||
Internet Quality |
Average Internet speed (Mbps) |
||
Popularity |
Google search trends index score |
||
Diversity and |
Student Diversity |
Percentage of foreign students in higher education |
|
Political Participation |
Voter turnout |
||
Demographic Diversity |
Non-citizens’ share of the total population |
Cities Included in the Index
Region | Cities |
Africa | Cairo, Casablanca, Johannesburg, Lagos, Nairobi |
Western Europe | Amsterdam, Athens, Berlin, Brussels, Copenhagen, Lisbon, London, Madrid, Paris, Rome, Stockholm, Vienna |
Eastern Europe | Belgrade, Budapest, Moscow, Prague, Sofia, Warsaw |
South America | Buenos Aires, Rio de Janeiro, Santiago |
South and Southeast Asia | Bangkok, Delhi, Dhaka, Jakarta, Karachi, Kuala Lumpur |
North America | Chicago, Mexico City, New York, Toronto |
Middle East | Ankara, Antalya, Beirut, Dubai, Istanbul, Izmir, Konya, Riyadh, Tehran, Tel Aviv |
Central and East Asia | Almaty, Baku, Beijing, Hong Kong, Seoul, Shanghai, Tokyo |
Oceania | Auckland, Sydney |
Because the main approach of the City Development Index involves the availability of statistical quantitative data, the data collection phase directly affected the indicator construction process. The standardization, winsorization, normalization, and imputation stages occurred in that order once the raw data had been collected; thus, the indicator scores required for weighting were formed by processing the raw data.
Through about 10 months of meticulous work, the project team obtained the national and international raw data within the scope of the City Development Index from the databases of international organizations such as the UN, OECD, ILO, World Bank, UNESCO, Eurostat, as well as from the statistical offices of countries and cities. The first stage standardized the raw data for all the cities by converting the data to the same units of measurement (e.g., percentages, quantities, per capita values).
In order to prevent outliers in each indicator from dominating the index results, the second stage determined indicators with skewness values greater than 2 and kurtosis values greater than 3.5, then the outliers in these indicators were winsorized to the nearest maximum or minimum value.
The third stage normalized all indicators using the method of adjusting to the maximum value in order to ensure that the indicators with different measurement units are on the same page and to avoid over- or under-representation in the index score. Thus, values were produced in the range of 0-100 for each indicator. The advantage of adjusting to the maximum value is that the pre-normalized structure of the data remains intact after normalization. Other normalization methods partially disrupt the structure of the data, narrowing distributions with wide ranges and widening distributions with narrow ranges. After normalization, negative indicators were subtracted from 100, and the direction of the indicator was reversed.
The fourth stage estimated the missing data for each indicator using imputation techniques when no data were available for a city or when deficiencies occurred in the temporal data of a city. In the case of city data being absent for certain years, the values for the missing years were estimated according to the structure of the data using a projection method by averaging the previous and following years or by using the most recently published data. If no data could be found for a city specific to that indicator, the city data was obtained by taking a relative proportion from the region and country data. If country data were unavailable, the missing data were completed by taking the value as the average value of the dimension included in that indicator.
After completing the data processing phases, the indicators were weighted and combined with the dimensions. The criteria importance through intercriteria correlation (CRITIC) method was used to weight the indicators. With this method, weighting is done according to two basic criteria: the first is the standard deviation value, which shows the spread of the observed values for the variable, and the second is the correlation coefficients, which shows the intensity of the relationships among variables. Accordingly, the weight of a variable is directly proportional to the standard deviation of that variable and inversely proportional to the correlation value between it and the other variables. The final stage combined the indicators using the weighted arithmetic average method while calculating the index score. Thus, the weight of a dimension was obtained by adding the weights of the indicators making up that dimension, and the weight of a domain was obtained by adding the dimensions that make up that domain.
The final stage also calculated the dimension, domain, and indicator scores for each city between 2010-2020 and ranked the cities individually according to the calculated indicator, domain, and dimension scores. Thus, the cities at the top, bottom, and middle were identified with respect to their dimension, domain, and index scores. The cities and nine regions where these cities are located were evaluated with respect to their 11-year average scores. As a result of the rankings, the cities were additionally classified in different categories with respect to both the rise and fall in rank over the years as well as to their average rank.
The Direction the Indicators Contribute to the Index, and Weightings for the Indicators, Dimensions, and Domains
INDICATORS |
INDICATOR WAY |
INDICATOR WEIGHT |
DIMENSION WEIGHT |
DOMAIN WEIGHT |
Family Sustainability |
Positive |
2.11% |
8.26% |
37.18% |
Population Reproduction |
Positive |
1.50% |
||
Life Expectancy |
Positive |
0.51% |
||
Infant Mortality |
Negative |
2.15% |
||
Age Dependency |
Negative |
2.01% |
||
Poverty |
Negative |
1.96% |
8.33% |
|
Automobile Ownership |
Positive |
2.23% |
||
Cost of Living |
Negative |
1.91% |
||
Comfortable Public Transportation |
Positive |
2.22% |
||
Access to Health |
Positive |
2.05% |
11.81% |
|
Traffic Accident Fatalities |
Negative |
2.48% |
||
Work Safety |
Negative |
2.02% |
||
Suicide |
Negative |
2.41% |
||
Intentional homicides |
Negative |
2.85% |
||
Recycling |
Positive |
1.86% |
8.79% |
|
Air Quality |
Negative |
2.37% |
||
Electricity Consumption |
Positive |
2.23% |
||
Water Consumption |
Positive |
2.34% |
||
GDP Per Capita |
Positive |
1.87% |
8.23% |
32.37% |
Income Equality |
Negative |
1.48% |
||
Household Debt |
Negative |
2.44% |
||
Inflation |
Negative |
2.43% |
||
Investment |
Positive |
1.62% |
6.86% |
|
Economic Transformation |
Positive |
1.31% |
||
Female Labor Force Participation |
Positive |
1.76% |
||
Taxation |
Positive |
2.16% |
||
Diversity in Work Life |
Positive |
3.17% |
10.76% |
|
Foreign Direct Investment |
Positive |
2.63% |
||
Economic Connection |
Positive |
2.63% |
||
Foreign Trade Balance |
Positive |
2.34% |
||
Employment Structure |
Positive |
1.20% |
6.52% |
|
Labor Force Participation |
Positive |
1.04% |
||
Unemployment |
Negative |
2.42% |
||
Qualified Labor |
Positive |
1.86% |
||
Enrollment |
Positive |
0.89% |
4.35% |
30.45% |
Population in universities |
Positive |
2.05% |
||
Quality of Education |
Negative |
1.40% |
||
Population with Higher Education |
Positive |
1.73% |
8.80% |
|
Number of Researchers |
Positive |
2.25% |
||
R&D Expenditures |
Positive |
1.97% |
||
Intellectual Property |
Positive |
2.84% |
||
Connectivity |
Positive |
2.37% |
9.57% |
|
Attractivity |
Positive |
2.67% |
||
Internet Quality |
Positive |
1.96% |
||
Popularity |
Positive |
2.55% |
||
Student Diversity |
Positive |
2.56% |
7.74% |
|
Political Participation |
Positive |
2.28% |
||
Demographic Diversity |
Positive |
2.90% |